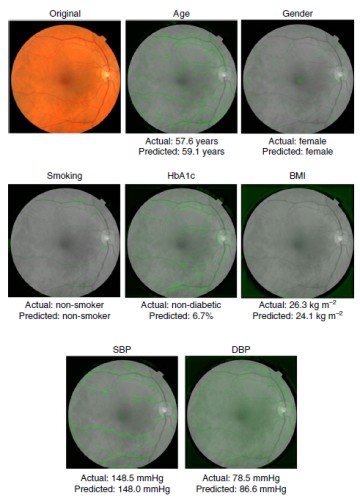
Scientists from Google and its subsidiary Verily, which specializes in the development of medical technology, have developed a new way to identify risk factors for the development of cardiovascular diseases, such as coronary heart disease and stroke. A trained neural network rather accurately calculates these factors using the fundus image.
The photo in the upper left corner shows a color sample of the fundus scan from the UK Biobank database. The remaining images are represented by the same image, but in black and white. On each of them a green map is applied, corresponding to each of the learned signs: age, gender, smoking (yes / no), average blood sugar HbA1c, body mass index BMI, arterial systolic pressure SBP, arterial diastolic pressure DBP. The real data from the database for each parameter and predicted by the neural network are indicated.
Knowing these factors, one can quite accurately calculate the probability of developing cardiovascular diseases, which are the
main cause of death worldwide (about 31% of deaths are caused by this very reason).
With the help of the new system, doctors can save a lot of time, because instead of several tests now preliminary diagnosis is performed in a few minutes. Moreover, theoretically, the algorithm allows such diagnostics to be carried out remotely. All you need is an ophthalmoscope and a specialist who can take a picture.
Mirror and electronic ophthalmoscopesOf course, while the accuracy of the neural network is not so high as to replace a full-fledged diagnosis, it does show promising results. Here AI does not replace the doctor, but expands its capabilities.
Scientists at Google and Verily have used medical records with photos of the fundus of approximately 300,000 patients to train the neural network. The lion's part of the data set was obtained from the EyePACS database (236,234 patients, 1,682,938 images). The rest of the information was taken from the UK Biobank database. Although there is less data volume, then for each patient there was information on body mass index, blood pressure and the fact of smoking, which is not in the EyePACS database.
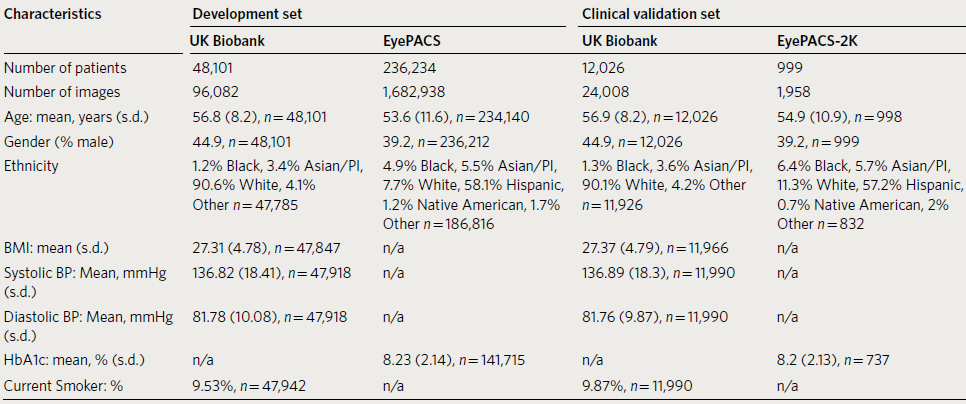
The idea of identifying human diseases through the retina is not new. Back in the Soviet Union, such studies were carried out and software for image analysis of the retina was created. But then there were no machine learning systems, so the possibilities of programmers were limited.
If Google’s neural network receives two patients for processing the fundus photo, one of whom has suffered from a cardiovascular disease in the past five years and the other doesn’t, then it correctly identifies the photo to the patient in 70% of cases. This is slightly worse than the accuracy of the SCORE algorithm currently used in medicine. He has an accuracy of 72%.
The accuracy of determining gender, age and each risk factor is shown in the following table.

Experts say that Google’s approach to the use of a neural network in this particular diagnostic task inspires confidence because it has long been known that the retina of the eye well predicts the risk of developing cardiovascular diseases. So, artificial intelligence can significantly speed up, and potentially increase the accuracy of such diagnostics. Of course, before real use in clinics, the program must be thoroughly tested so that doctors begin to trust it.
This discovery was another proof that neural networks can be widely used in modern medicine, especially in diagnostics. We are only groping for the most obvious options for applying AI in this area:
diagnosis of arrhythmias from a cardiogram ,
diagnosis of pneumonia from X-rays ,
diagnosis of skin cancer , etc.
The tremendous use of AI for diagnosing diseases is one of the reasons why Google launched
the Baseline project to collect detailed medical records of 10,000 people over four years.
The scientific article was
published on February 19, 2018 in the journal
Nature Biomedical Engineering (doi: 10.1038 / s41551-018-0195-0,
pdf ).